Lei feng's network (search for "Lei feng's network" public attention) by writer Deng Qili, second-year computer Department of Harbin Institute of technology Shenzhen Graduate School, graduate, tutor for "city of scholars," Professor Xu. Research interest for deep learning and computer vision. First was awarded the 2015 Alibaba large-scale image search contest second prize, it ranked third.
Summary
In recent years, the depth of learning in computer vision tasks, have made a major breakthrough, one of the important factors is the strong nonlinear capabilities to understand the image deeper information. Based on deep learning Visual examples of the simple search method summarized and generalized, hopes to inspire readers. Fendi
Objective FENDI iPhone 6+
Given a query containing an object picture, Visual Search task is an instance found in the photo gallery from the candidate those queries image contains a picture of the same object. Compared with the General image search, instance search search criteria more demanding--contain the same objects, like a dress, the same car, and so on. The issue has a very wide range of applications, such as product search, vehicle search and location based on image recognition and so on. For example, mobile image search is through the analysis of goods using mobile phone camera photo, find the same or similar items from the library.
However, in the actual scene, because of the attitude, interference factors such as lighting and background, so the two images that contain the same objects tend to vary greatly in appearance. From this point of view, the Visual examples should search for the essence of the question is what kind of image feature which contains images of the same object is similar in feature space.
In recent years, deep learning in computer vision tasks are achieved major breakthroughs, including Visual examples search tasks. Paper main on based on depth learning of instance search algorithm (following referred to for "depth instance search algorithm") for analysis and summary, articles is divided into four a part: first part summary has classic Visual instance search algorithm of general process; second part and third part respectively from two a aspects to introduced in recent years main of depth instance search algorithm; end to end of features learning method and based on CNN features of features coding method Part I will summarize in 2015 first Alibaba a large image competition (Alibaba Large-scale Image Search Challenge, ALISC) appears in the related methods, introduce some examples of practice can improve search performance techniques and methods.
Classic examples of Visual search algorithm flow
In depth learning popular zhiqian, typical of instance search algorithm General is divided into three a stage: first in given of image intensive to extraction local not variable features, then will these local not variable features further to coding for a compact of image said, last will query image and candidate image library in the of image for similar degrees calculation (based on second step get of image said), found those belongs to same instance of pictures.
1. local invariant features. Extracting local invariant features details about local area of an image, do not care about the global information, and light within the local area has some invariant, geometric transformation. This for instance searching makes perfect sense because the target objects can be accompanied by a geometric transformation occurs in any area of an image. In earlier work in many instances search method using SIFT features.
2. character encoding. Significance to local features further coding is two-fold: mining information between these local features, enhanced discrimination ability; a single compact index of the eigenvectors is easier to implement, improve search speed. Common methods of VLAD (vector of locally aggregated descriptors), Fisher Vectors,triangular embedding. In here, paper simple to introduced Xia VLAD method (in paper behind times appeared): a,) VLAD method first using k-means get contains k a center of code this, then each local features was assigned to away from it recently of Center points (we will this a step called hard-assignment, zhihou will related articles on this for improved), last will these local features with assigned of Center points Zhijian of residual poor tired and as eventually of image said. From above we can see that VLAD disorder – don't care about local properties of spatial location, so you can further decouple global space information, is very robust to geometric transform.
3. the similarity calculation. A direct approach is based on distance function calculates the distance between features, such as the Euclidean distance, cosine distance. Another is learning the appropriate distance function, such as LMNN, ITML and measurement methods.
Summary: classic examples of Visual search algorithm performance is limited by the characteristics of hand-crafted said. In instances when the application deep learning search tasks, mainly feature from the start, namely how to extract more distinguishing images features
Characteristics of end-to-end learning method
NetVLAD: CNN architecture for weakly supervised place recognition (CVPR 2016)
This article is from INRIA Relja against Arandjelovi and other people's work. The article concerns a specific application instance search--location awareness. Position recognition problem, given a query image by querying a large place markers for a data set, and then use those similar picture to evaluate queries image locations. First, using Google Street View where Time Machine for mass tagging data set, then presented a Convolutional neural network architecture, NetVLAD--, VLAD method embedded in the CNN network, and "end-to-end" of learning. The method shown in the following figure:
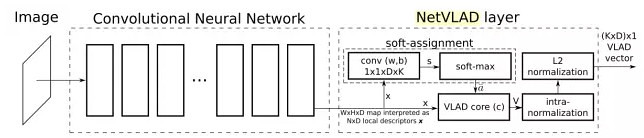
Hard-assignment is not in the original VLAD micro (local characteristic of each assigned to its nearest the center point) and therefore cannot be directly embedded into the CNN network, and participate in the error back-propagation. This article's workaround is using the softmax function to the hard-assignment operation soft-assignment operation-use 1x1 convolution and softmax function to get the local feature belongs to probability/weight of each center point, and then assigns maximum probability weight/center point. Therefore NetVLAD contains three parameters can be studied, and 1x1 above which is the convolution of the parameters used to predict soft-assignment, expressed as the center point of each cluster. And then on the figure of VLAD in the core layer through the accumulation of residual action. Author gives us the following figure illustrate the advantages of NetVLAD compared to the original VLAD: (more flexibility--learn better cluster-center point)

Another improvement for this article is Weakly supervised triplet ranking loss. The method to solve the problem of training data may contain noise, triplet ranking replace respectively with potential loss of positive and negative samples are samples (containing at least one sample, but not sure which one) and a clear set of negative samples. And when you train, bound queries image and sample concentration is most likely are samples of the characteristic distance between pictures than queries image with all negative characteristic distance between pictures in the sample set is smaller.
Deep Relative Distance Learning: Tell the Difference Between Similar Vehicles (CVPR 2016)
Next article focused on vehicle identification/search problem, from Hongye Liu, who works at Peking University. As shown in the following figure, this problem can also be seen as instances search tasks.

And a lot of the depth of supervisory instance search methods, this article is an attempt to map the original picture to a European in the feature space, and makes the space, pictures gathered more of the same vehicle, rather than a similar vehicle pictures are even more far away. In order to achieve the effect, the commonly used method is by optimizing the triplet ranking loss, to train the CNN network. However, the authors found that the original triplet ranking loss, there are some problems, as shown in the following figure:

For the same sample, triples will be on the left of the loss function adjustment and the triple to the right is ignored. Difference between the two lies in the choice of anchor is different, this led to instability while training. In order to overcome this problem, by using coupled clusters loss (CCL) to replace the triplet ranking loss. The loss function is characterized by the triples into a sample set and a set of negative samples and samples gathered in the sample, samples of negative samples in the sample set and those who are further apart, so as to avoid the negative impact of randomly selected samples of anchor. The effect of the loss function as shown in the following figure:
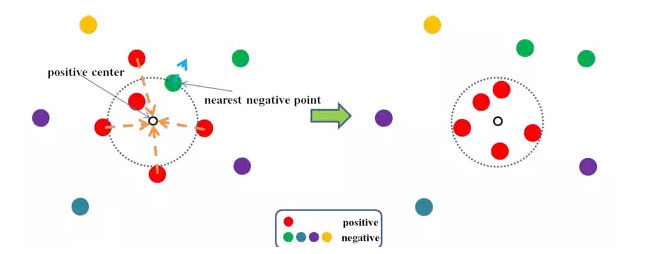
Finally this article for the particularity of vehicle problems, and design coupled clusters above loss, a hybrid network architecture is designed, and built a vehicle database so as to provide the necessary training.
DeepFashion: Powering Robust Clothes Recognition and Retrieval with Rich Annotations (CVPR 2016)
Finally, this article was also published in CVPR 2016, clothes were introduced to identify and search, also is an instance with search-related tasks from the Ziwei Liu, who works at the Chinese University of Hong Kong. First of all, this article introduces a database of clothes called DeepFashion. The database contains over 800K dress pictures, 50 fine grained categories and 1000 properties, and can also provide an additional key to clothes clothes attitude/cross-cutting and cross-relationship (cross-pose/cross-domain pair correspondences), some specific examples shown in the following figure:
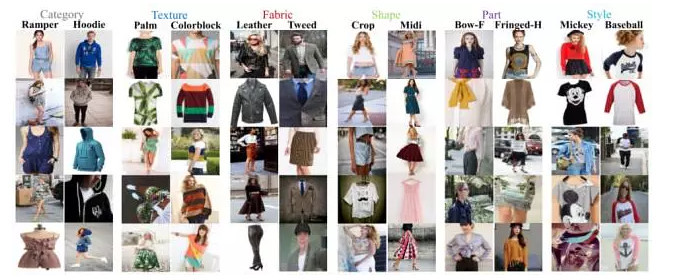
In order to illustrate the effect of the database, the author presents a new depth of learning networks, FashionNet--through the key points of the joint forecast clothes and property, learning to be more differentiated features. The overall framework of the network looks like this:
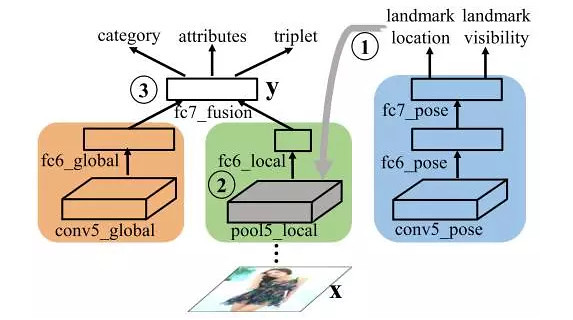
FashionNet forward the calculation process is divided into three phases: the first phase, a clothes picture blue branch of the input to the network, the key to predicting clothes are visible and the position of the point. The second phase, according to the forecast in the previous step's key points, the key pool (landmark pooling layer) get clothes of local features. The third phase, "FC6 global" global features and "FC6 local" local features are spliced together to form a "fc7_fusion", as the final image. FashionNet introduces four loss function, and uses an iterative training to optimize. These losses are as follows: return loss corresponds to a key position, softmax loss is visible corresponds to the key points and clothing category, cross-entropy loss function corresponds to property prediction and triples loss function corresponds to the similarities between the clothes. Authors from the clothing category, property prediction and clothing searches these three aspects, FashionNet compared to other methods, have achieved significantly better results.
Summary: when there are enough tagging data, deep learning can learn features and measurement functions. The idea behind it is based on a given metric, learning characteristic features in the metric space has the best judgement. End-to-end feature learning characteristics of main research interest is how to build a better representation and loss of function.
CNN features the character coding method
Depth of instances of the search algorithm described in the above section of this article, and focuses on data-driven end-to-end characteristics of learning methods and corresponding images to search for data sets. Next, we focus on another issue: when there is no search data related to these sets, how to extract a valid image characteristics. In order to overcome the lack of field data, pre-training model is a viable strategy in the CNN (CNN model of training data sets in other tasks, like ImageNet taxonomy data set) based on extracting characteristics of a layer map (feature map), encode it to be suitable for instance search feature. This section under related papers in recent years, some of the key methods (in particular, all CNN model in this section are based on the ImageNet set of training models for categorical data).
Multi-Scale Orderless Pooling of Deep Convolutional Activation Features (ECCV 2014)
This article was published in ECCV 2014, is from the University of North Carolina at Chapel Hill Yunchao Gong and others, including the University of Illinois at Urbana-Champaign Liwei Wang's work. Because the CNN feature missing geometric invariance limits the variable scene classification and matching. Author attributed the problem of global CNN feature contains too much information and therefore made a multi-scale orderless pooling (MOP-CNN)-CNN features VLAD method combined with the disorder.
MOP-CNN the main steps, CNN network is first seen as a "local character" extractor and extract image on multiple scales "local character", and with VLAD the size of each "feature" encoding for the scale image features, and finally all the scale image features to connect together to form the final image. Feature extraction framework as shown below:
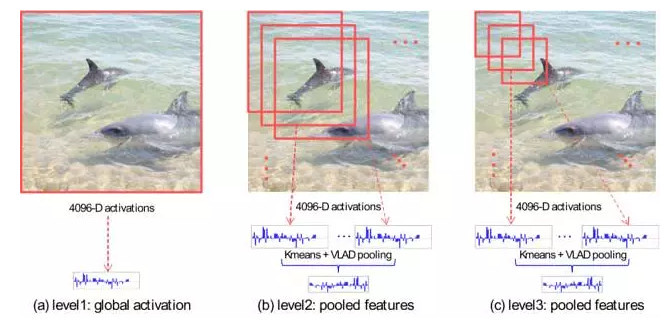
Author search on the classification and case test on two tasks, as shown in the following figure, proves that MOP-CNN than in a regular CNN global features a better classification and search results.
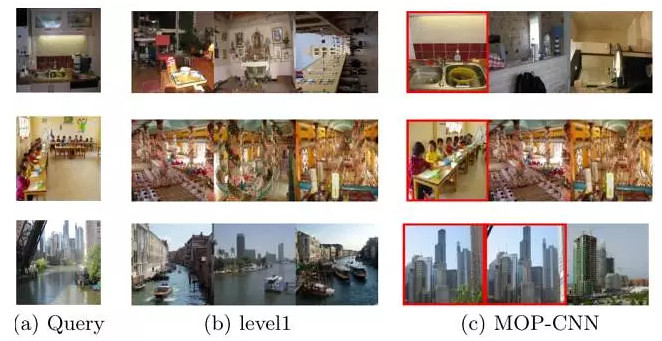
Exploiting Local Features from Deep Networks for Image Retrieval (CVPR 2015 workshop)
This article was published in a CVPR 2015 on the workshop, from the University of Maryland, College Park people such as Joe Yue-Hei Ng. Recent studies showed that, compared to the linked layer's output, convolution of feature maps (feature map) is more suitable for instance search. This article describes how to use the convolution of feature maps into a "local character", and VLAD the encoding to use for the image feature. In addition, the authors also conducted a series of experiments to observe different convolution effect of signatures for instance search accuracy.
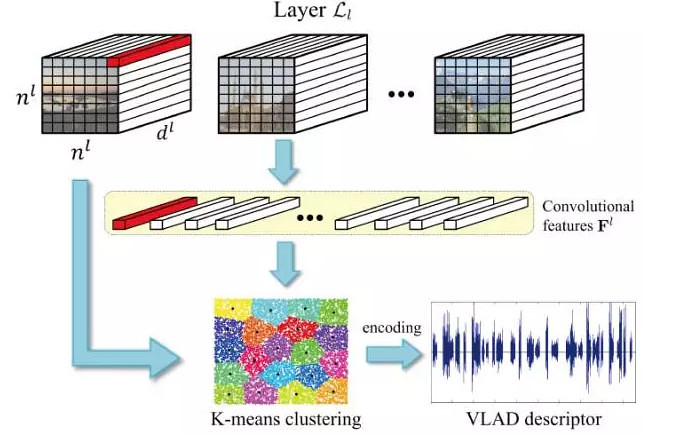
Aggregating Deep Convolutional Features for Image Retrieval(ICCV 2015)
This article was published on the ICCV 2015 next, comes from the Moscow Institute of physics and technology Artem Babenko and Victor Lempitsky skolkovo technology college work. Can be seen from the above two articles, a lot of depth examples search method using chaotic-coded method. But these, including the VLAD,Fisher Vector coding method of computation is usually relatively large. In order to overcome this problem, this article we design a simpler and more efficient coding method--Sum pooing. Sum of pooling specific definition looks like this:

Which is the convolution in the spatial location of local features (local feature extraction methods, consistent with the last article). Using sum after pooling, global features to further implementation of PCA and L2 normalized to get the final features. Fisher Vector,Triangulation embedding and author Max pooling and comparing these methods proved sum pooling method is not only simple, and better.
Where to Focus: Query Adaptive Matching for Instance Retrieval Using Convolutional Feature Maps (arXiv 1606.6811)
Finally this article posted on the arXiv, came from Australia, University of Queensland Jiewei Cao and other people's work. As mentioned at the beginning of this article, messy background for instance search is very large. In order to overcome this problem, this article based on the article on the method of sum-pooling, presents a known query Adaptive matching (QAM) methodology to calculate the similarity between images. The core of the method is to perform pooled operations on multiple areas of an image, and create more characters to express images. And then in the match, queries image will correspond to the characteristics of these regions and best match score as a similarity between two images. So the next question is how to build this area.
Author proposes two approaches--Feature Map Pooling and Overlapped Spatial Pyramid Pooling (OSPP), to get the base region of the image. And then through the base region continue to merge, in order to find the best similarity scores for goals, build out of the goal area. One of the most attractive part is that authors will throughout the merger process, into the solution of a problem. Graph below shows part of QAM method results and the corresponding image map.
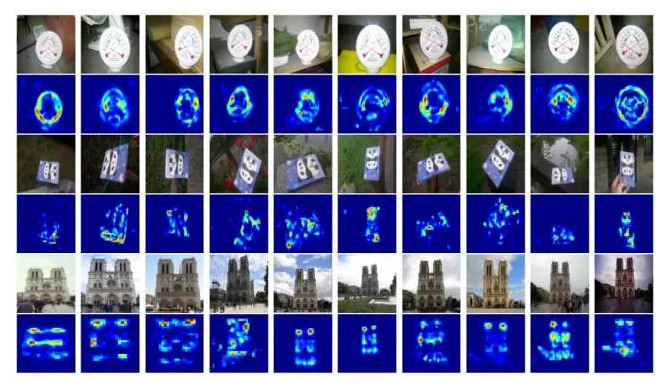
Summary: in some instances the search task, due to the lack of sufficient training, so you can't directly "end to end" to learn of image features. At this time, how to turn ready-made CNN feature coding for instance Search images that become a hot research area.
2015 first summarises Alibaba a large image contest
Introduction in recent years, some examples of the depth of the main search method when you are finished, in the next part of this paper will summarize in Alibaba a large search contest related methods that appear in the image, to introduce some practice, you can improve the performance of Visual examples search techniques and methods.
Large-scale image search image search contest by Ali Alibaba group sponsored by requiring participating teams from huge Photo Gallery to find out those queries image contains a picture of the same object. This competition provides the following two types of data used for training: training about 200W picture collection (category levels that correspond to the labels and attributes), 1417-verify queries image and the corresponding search results (for a total of approximately 10W). In tests, given 3567 queries image, teams need from approximately 300W picture evaluation focused (no label), pictures of people who meet the requirements, evaluation is based on the top 20 of the mAP (mean Average Precision).
Briefly introduce our method for--Multi-level Image Representation for Instance Retrieval, which made the third. Many methods are used at the end of a convolution or retrieves the link layer characteristics, due to the high level of features have lost a lot of detail (for a deeper network, more serious loss), so the instance search is not very accurate, as shown in the following figure, the overall outline is similar, but the details are far.
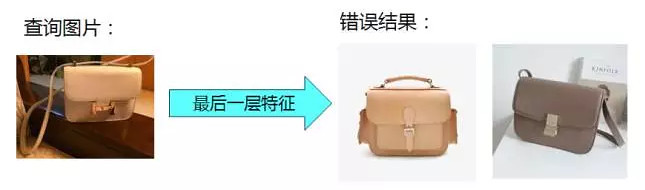
In order to overcome this problem, we will feature maps of the different layers in the CNN network (feature map) for integration, not only using the high-level features of semantic information, as well as details of the low-level features information, instance search more accurate. As shown in the following figure, our experiment is based on the GoogLeNet-22 network, for the last 8 characters (from the Inception of 3B to Inception 5B), first use the largest pool of these feature maps of different scales are sampled (converted to a character of the same), and use of these sampling results further convolution. And then these characteristics make weighted linear (convolution), on the basis of using sum pooling to get the final image. In training, we are based on the training data provided by optimizing triplet based on cosine distance ranking loss to end-to-end learning these characteristics. During testing, you can directly use cosine distances between features to measure the similarity of the image.
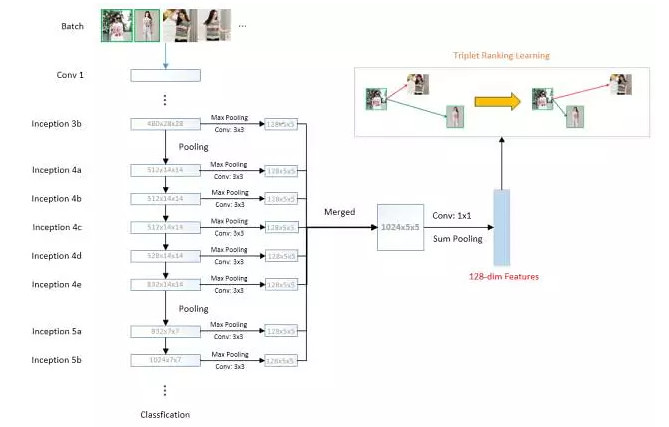
Addition, reference Yu training SVM classification device Shi using has difficult points sample mining of thought, we of method first in Qian to calculation Shi, calculation current training batch in the all potential ternary group of loss (from current training batch in the selected two Zhang same category of pictures and a Zhang different category of pictures constitute potential ternary group), then found those "difficult" of ternary Group (more big of loss), last in reverse calculation Shi, using these "difficult" of ternary group for errors spread, to made better of training effect.
Next a brief summary of related methods that other teams. Characteristics of end-to-end learning methods, in addition to triplet ranking loss,Contrastive loss (corresponds to the Siamese network) is also a common loss function. In addition, there are worthy of our attention, you can improve search performance dramatically:
(A) mining
In supervised machine learning methods, more data can mean a higher degree of accuracy. Team in the calculations come from the Chinese Academy of Sciences, under the ImageNet-feature of the training model, clustering on the training set at the category level, and then through the threshold, more out with the figure, and with plans to train in the CNN network, learning feature. This method is simple, and you can improve search performance dramatically.
(B) target detection
Case retrieval in the complex final search performance is directly affected by the background noise. First, so a lot of teams try to use object detection (such as faster-rcnn) to locate areas of interest, and then further learning features, compare similarities. In addition, when there are no bounding box when training data, weak supervision is an effective method to locate the target.
(C) the first pool features and erjiechi characters fusion
Erjiechi method of capturing images of second-order statistical variables, covariance, can often achieve better search accuracy. Team led by Professor Li Peihua from Dalian in the CNN network based on the characteristics of first-order characteristics of pools and erjiechi integration, achieving very good results.
(D) joint feature and property prediction
This method and the DeepFashion mentioned in the third part of this article is similar to learning characteristics and prediction of properties of the picture (missions), resulting in more differentiated features.
Lei Feng network Note: authorized to Lei feng was released by deep learning Forum in this paper, please indicate the author and the source, no deletion of content.